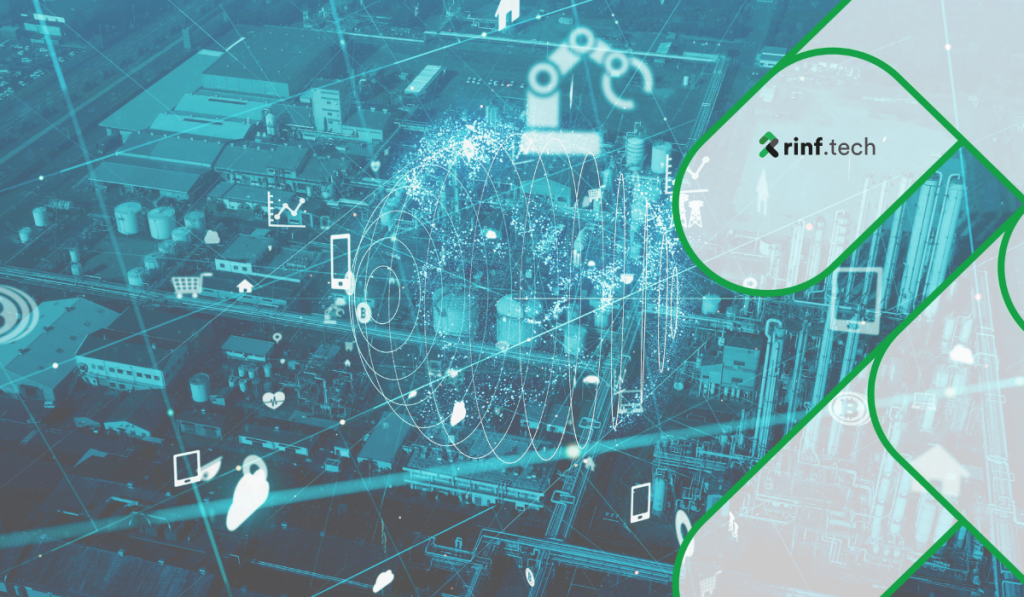
IoT Device Onboarding Requirements, Challenges, and Best Practices
Delving into the IoT device onboarding requirements, challenges and implementation best practices across smart home, industrial, and healthcare settings.
According to Precedence Research, the global Industrial IoT market size is expected to reach around $1,562.35 billion by 2032. The IIoT market’s growth is driven by the convergence of several pivotal factors that are reshaping the manufacturing landscape.
Gone are the days when machinery maintenance depended solely on scheduled inspections or reactive measures following equipment breakdown. Adopting predictive maintenance practices represents a paradigm shift, where data-driven insights guide the timing and extent of maintenance interventions.
This article will explore how IIoT facilitates predictive maintenance and discuss its essential components and implementation challenges and considerations.
Predictive maintenance utilizes real-time data and analytics to predict when an equipment failure might occur, allowing for timely intervention. In contrast, preventative maintenance is scheduled at regular intervals regardless of the actual condition of the machine. This proactive approach ensures maintenance is performed only when necessary, reducing costs and downtime.
The ability to accurately predict equipment failures not only minimizes operational disruptions but also leads to more effective resource allocation. In a world driven by efficiency and sustainability, predictive maintenance serves as a cornerstone for competitive advantage. It enables organizations to improve productivity, achieve regulatory compliance, and maintain a high-quality standard.
A comprehensive predictive maintenance system includes sensors for data collection, data analytics platforms for interpretation, and user interfaces for decision-making. The sensors monitor various indicators like temperature, vibration, and pressure, among others. The analytics platform uses machine learning algorithms to analyze the collected data and make predictions, while the user interface displays actionable insights.
The advent of the IIoT has taken predictive maintenance to unparalleled levels of sophistication and effectiveness. Fundamentally, IIoT infuses industrial settings with interconnectedness, enhanced data gathering capabilities, and sophisticated analytical tools. This convergence enables more insightful decision-making, the anticipation of maintenance needs, and the streamlining of operations.
One of the foundational elements of a successful IIoT predictive maintenance system is effective IoT device management. Efficient device management ensures that each connected device operates optimally, receives timely updates, and functions in harmony with other devices in the ecosystem. This coordination is vital as the sheer number of devices in large-scale industries can be overwhelming, and proper management is necessary for the system’s effectiveness to be maintained.
The rise of digital twins represents a cutting-edge milestone within the IIoT realm. A digital twin serves as a dynamic, digital avatar of a physical asset, delivering enhanced diagnostics and prognostics prowess through live data streams and advanced modeling techniques. By mirroring its physical counterpart in the digital ecosystem, a digital twin can forecast potential issues or inefficiencies, enabling preemptive action. This capability dramatically reduces downtime and fine-tunes performance by running simulations across a spectrum of scenarios and proposing actionable refinements, particularly in the context of robotic armature.
Our team at rinf.tech had the opportunity to develop a digital twin system that would enable remote control of our universal (UR3) robots. The cutting-edge system allows users to easily upload a 3D model of the robot and modify its dimensions and location in a physical space for the best possible user experience. The virtual robot can be manipulated with natural hand gestures, and the physical robot will imitate it. Easy button interactions are made possible by a user-friendly menu tethered to the left hand and triggered by facing the right palm inward for detailed customization.
IIoT uses a wide range of equipment and sensors, including ultrasonic detectors, accelerometers, and temperature sensors, to mention a few. Numerous characteristics, including but not limited to temperature, vibration, pressure, and sound levels, can be measured by these sensors. When these sensors are combined with edge computing capabilities, they can process raw data first and then send the cleaned data to the cloud for additional analysis.
Temperature Sensors: These are crucial for machines that are sensitive to temperature fluctuations. Overheating is often a sign of malfunction or impending failure.
Vibration Sensors: Often used in rotating machinery like turbines and compressors, these sensors can detect imbalances or misalignments before they become critical.
Acoustic Sensors: These can pick up ultrasonic noises emitted by failing equipment, which are inaudible to the human ear but indicative of issues like gas leaks or electrical discharge.
Pressure Sensors: Crucial in hydraulic systems, these sensors monitor fluid pressure to ensure it remains within operational limits.
Humidity Sensors: In environments where moisture levels are critical, such as food processing or pharmaceuticals, these sensors can provide valuable data.
Flow Meters: Used extensively in liquid processing industries, these devices can identify irregularities in the flow rate, which may signify problems in the system.
Electrical Current Monitors: These can detect anomalies in electrical consumption, signaling issues like overloads or potential circuit failures.
Once the IIoT devices collect the data, it is transmitted to a centralized database, often in the cloud but sometimes on-premises, based on the specific requirements of the business. The data is then processed and analyzed using advanced analytics platforms that employ machine learning algorithms and data science techniques.
These algorithms are trained to recognize complex patterns and trends within the data, which human analysts might find difficult to spot. By learning from historical machine behavior and real-time data, the system can identify anomalies symptomatic of mechanical problems. When such abnormalities are detected, alerts are generated to notify the maintenance teams.
Additionally, the predictive maintenance system may also provide detailed recommendations on what corrective actions should be taken, whether it’s a minor adjustment or a more involved part replacement. This ensures that not only is the problem identified in advance, but it can also be accurately diagnosed and fixed before it leads to a costly shutdown.
The cost-saving potential of IIoT-enabled predictive maintenance is one of its most compelling benefits. Traditional maintenance methods often lead to over-maintenance, where machinery is routinely checked and serviced even when it doesn’t need to be, or under-maintenance, where failures are dealt with as they occur. Both approaches come with significant costs. Predictive maintenance reduces the need for unnecessary maintenance tasks, thus saving labor time and parts.
Moreover, downtime, particularly unplanned downtime, can have a devastating impact on a business’s revenue and reputation. Predictive maintenance identifies the likelihood of equipment failure ahead of time, allowing companies to perform maintenance activities during non-productive hours, thereby minimizing operational disruptions and avoiding financial losses associated with downtime.
IoT-enabled predictive maintenance also contributes to the extension of asset lifetimes. A well-maintained machine suffers less wear and tear, operates at peak efficiency for longer periods, and generally has a longer lifespan compared to a poorly maintained one. This not only saves costs but also reduces the frequency of capital expenditure required to replace machinery and equipment.
The advanced sensors and algorithms can also monitor other factors contributing to asset deterioration, like environmental conditions and operational pressures. By understanding and controlling these variables, companies can make data-driven decisions that contribute to extending asset life further, making it a sustainable option in the long term.
Safety is an often overlooked but significant benefit of predictive maintenance. Malfunctioning equipment is more than just an operational issue. It’s a safety hazard. Whether it’s a piece of machinery in a manufacturing plant or a component in an electrical grid, failures can have serious safety implications, including workplace accidents or catastrophic events.
IIoT-enabled predictive maintenance provides real-time monitoring, which means it can alert operators to potential safety issues before they become critical. This enables proactive action to mitigate risks, thereby not only protecting the assets but more importantly, safeguarding human lives and the environment.
In traditional maintenance models, resource allocation is often a balancing act between over-provisioning and risking unavailability when needed. Predictive maintenance, enabled by IIoT, optimizes the use of maintenance personnel and resources by providing detailed information on equipment conditions. This ensures that resources are deployed where needed and that maintenance personnel are equipped with the knowledge to address issues effectively.
By optimizing resource allocation, companies can reduce overhead costs and improve the efficiency of their maintenance operations. For instance, predictive maintenance can reduce the need for emergency callouts, which are often more expensive than planned maintenance activities. It also enables better planning for inventory, meaning organizations can keep fewer spare parts on hand, reducing holding costs.
Data privacy and security are essential concerns when implementing any IIoT solution, and predictive maintenance is no exception. Potential cyber threats have a larger attack surface due to the growing number of connected devices. These security flaws could jeopardize not only the data integrity but also the equipment’s ability to function. Robust cybersecurity protocols, such as multi-factor authentication, end-to-end encryption, and frequent security assessments, are essential for companies.
One of the biggest challenges organizations face is the integration of new IIoT devices with existing legacy systems. Many industrial setups have been in operation for several years, even decades, and may not be inherently designed to accommodate modern IIoT technology. Upgrading these systems can be expensive, time-consuming, and occasionally require a total reconstruction of the current infrastructure.
Predictive maintenance can only be as effective as the data it uses. Inaccurate or low-quality data might result in forecast errors that require unnecessary maintenance or, worse, fail to identify a crucial problem. To guarantee that the data being examined is accurate and reliable, the maintenance plan should include procedures for sensor calibration, data cleansing, and validation.
Before committing to a specific predictive maintenance solution, decision-makers must evaluate how well it will integrate with their existing operational landscape. Assessments should include compatibility checks with existing hardware and software, as well as scalability tests to ensure the solution can adapt to future expansions or upgrades.
It might be challenging to calculate a predictive maintenance solution’s return on investment (ROI) but doing so is essential to supporting the original cost. The savings from longer asset lives, fewer downtimes, and more efficient use of resources should be compared to the system implementation and maintenance costs. Both concrete and intangible benefits will be included in a thorough ROI study, giving a more complete picture of the value created.
Implementing an IIoT-based predictive maintenance system is not just a technological shift but also a cultural one. It requires a skillset that may not be readily available in-house. Beyond the initial setup, staff members will need to be trained to interpret the data, operate the system, and make informed decisions based on the analytics provided. This requires investment in both time and training resources.
Before greenlighting an IIoT-powered predictive maintenance initiative, organizations must conduct a rigorous audit of their existing maintenance protocols and balance potential gains with strategic imperatives. It’s crucial for enterprises to quantify the frequency and fiscal fallout of equipment failures on their opex. A pivot to predictive maintenance is prudent if the data points to a significant bleed in resources due to maintenance snafus.
The enterprise’s tech stack must be primed for IIoT integration, assessing current capabilities to support this transition, particularly in the domains of network robustness and cybersecurity. It’s imperative to underscore the synergy between IIoT and the firm’s overarching tech roadmap. Predictive maintenance stands as a potent tool for amplifying productivity and safety metrics, while simultaneously curtailing operational costs.
A financial deep-dive is non-negotiable. The projected ROI must offset the upfront investment in IIoT enablement, factoring in not just the reduction in maintenance overhead and downtimes but also the adoption and operationalization expenses. An often-overlooked vector is the workforce’s aptitude to transition to a data-driven maintenance model; this may necessitate targeted upskilling initiatives.
Partner selection is another critical pivot — your choice should not only serve up a robust IIoT platform but also demonstrate a commitment to ceaseless innovation and support. Opt for a solution that’s both agile and scalable, one that’s built to scale with your enterprise and stay in lockstep with emergent tech.
Compliance with industry regulations and securing the IIoT ecosystem is paramount to sidestep legal pitfalls and safeguard the brand. Kick-starting with a pilot can distill insights to fine-tune strategies ahead of a broader rollout. Post-implementation, fostering a culture of iterative feedback is key to recalibrate the system and ensure the IIoT investment in predictive maintenance delivers optimal returns.
IIoT is at the forefront of a revolution in predictive maintenance, changing companies through a digital transformation wave sweeping across multiple industries. This paradigm shift isn’t just a technological advancement. It’s a comprehensive strategy for modern industrial operations that prioritizes proactive measures, data-driven choices, and optimization.
However, it’s important to underline that the transition to IIoT-based predictive maintenance is challenging. It needs a strategic vision, comprehensive planning, and the seamless integration of new technologies into existing operational frameworks. Beyond the technological considerations, there is a human element involved, requiring training, adaptability, and a willingness to evolve with the changing technological landscape.
Remaining ahead of the curve is not only beneficial but also essential for organizations seeking long-term success and resilience in this age of rapid technology breakthroughs. While implementing IIoT solutions is a positive step, selecting a partner for this venture is just as important.
We strongly urge technical decision-makers to prioritize quality, expertise, and innovation when selecting a provider for their IIoT needs.
As industries gear up for the future, making informed, forward-thinking decisions will be the cornerstone of sustained success and growth. Embrace the future, and let’s build it together.
Delving into the IoT device onboarding requirements, challenges and implementation best practices across smart home, industrial, and healthcare settings.
Delving into the complexities of AIoT, exploring its core principles, current state, challenges, and future trends.
Exploring how the key industrial IoT trends are poised to transform the future across multiple industries.