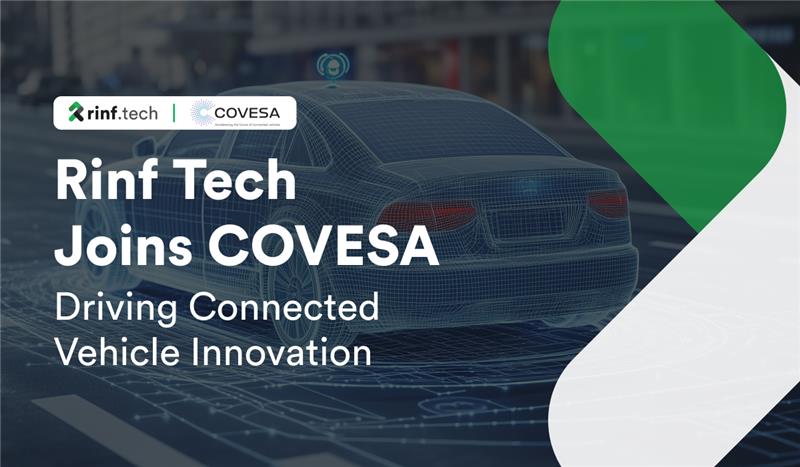
rinf.tech Joins COVESA to Advance Global Standards in Connected Vehicle Technology
We are proud to announce that rinf.tech has officially joined the Connected Vehicle Systems Alliance (COVESA). This milestone reinforces our commitment to open standards, cross-industry collaboration, and advancing software-defined vehicle technologies within the global automotive ecosystem.