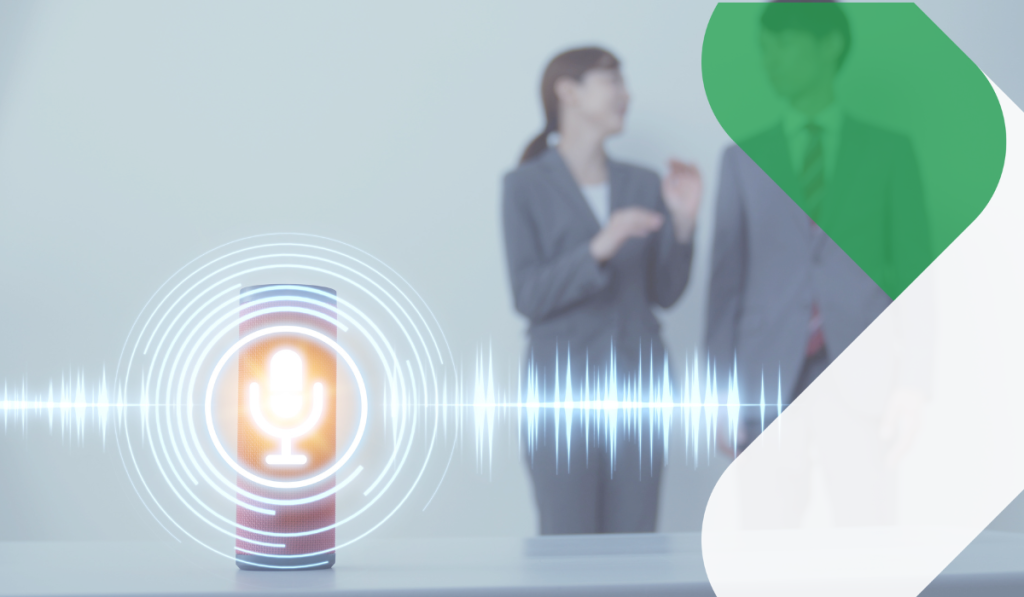
Voice Recognition and Security: Balancing Convenience and Privacy
Voice recognition technology is revolutionizing industries, from automotive and retail to fintech and healthcare, by enhancing user experience and operational efficiency. However, as these systems become more integrated into our daily lives, robust security measures are essential to protect sensitive voice data from emerging threats like spoofing and unauthorized access.